Geospatial Technology Takes on Cloud-Native Platforms
October 26, 2021
The geospatial technology sector has a problem. It’s data. As the geospatial industry developed in the late 70’s and 80’s, data storage and processing were becoming a big problem. DEC VAX microcomputers and later, IBM and Sun workstations became necessary for data computing needs. So, it’s no surprise today that the geospatial community always assumed we had a data problem. A “big data” problem.
The good news, however, is that in the intervening years, the sector has moved from simply collecting and creating huge data volumes to analyzing data. In addition, geospatial data constitutes a key data type in the broader information technology (IT) industry revealing insights that other data does not possess. As a result, advances in storage, processing, and machine learning, such as those offered by the current cloud data platforms, accommodate geospatial data types and the nuances created by collecting data from the Earth’s surface.
Data Warehouses or Data Lakes?
To users of geospatial information, storing and analyzing both unstructured and structured data leads to a discussion on how best to do both in a cloud-native environment. Organizations that currently leverage geospatial data have built data warehouses. This led to the creation of silos of each data type, which was proven to be an inefficient means for analytics. Some of these data are sourced from high velocity, real-time sensors that capture data about traffic, weather, water stream currents, and other sources, which became unusable within the architectural framework of data warehouses. As described by Databricks authors in a recent blog:
But while warehouses were great for structured data, a lot of modern enterprises have to deal with unstructured data, semi-structured data, and data with high variety, velocity, and volume. Data warehouses are not suited for many of these use cases, and they are certainly not the most cost efficient.
With this in mind, organizations began looking for a solution where many different geospatial-centric data types and formats could be stored in a single interoperable repository, and the concept of a data lake became popular.
This meant vector data such as KML, shapefile, WKT and GeoJSON, but also raster data like GeoTiff and other entities such as addresses needed to be geocoded.
Again, Databricks points to some challenges:
While suitable for storing data, data lakes lack some critical features: they do not support transactions, they do not enforce data quality, and their lack of consistency / isolation makes it almost impossible to mix appends and reads, and batch and streaming jobs. For these reasons, many of the promises of the data lakes have not materialized.
The switch from data warehouses to data lakes generated a new problem.
Deploying and managing these types of on-premise infrastructure and platforms used to take so much time from the Business and IT departments, that it left them very little time to leverage the platform and develop business use cases essential to adapt to the rapidly evolving business needs. Another solution had to be found.
Geospatial as Part of Mainstream IT
Within many organizations—whether the traditional government entities that employ large GIS departments or commercial companies that leverage location-based data for key business applications, such as insurance underwriting or retail site selection—geospatial data is frequently managed by central IT staffs. When talking about data governance for spatial data, an argument arises about whether the system of record should be the GIS platform that centralizes all geospatial data or the business system from where the data originated. Using the example of insurance, should the corporate IT system that manages policies and claims data that are geolocated by an address be the primary data store and hence be able to support geospatial data?
With that question in mind, geospatial software providers need to start adopting a “write once deploy anywhere” model. These solution providers must be able to deploy or integrate their capabilities outside of their centralized GIS platform and therefore geo-enable other applications. While geo-enabling business systems is already fairly common, geo-enabling the next generation platforms such as cloud-based big data analytics platforms is becoming a necessity, especially as location analytics become an important part of all analytics work. This is becoming essential, whether it’s a solution for big data analytics for large batch processing, or a large-scale, real-time integration that needs the high-availability and elasticity of a cloud-native Kubernetes cluster, for example. (See Figure 1)
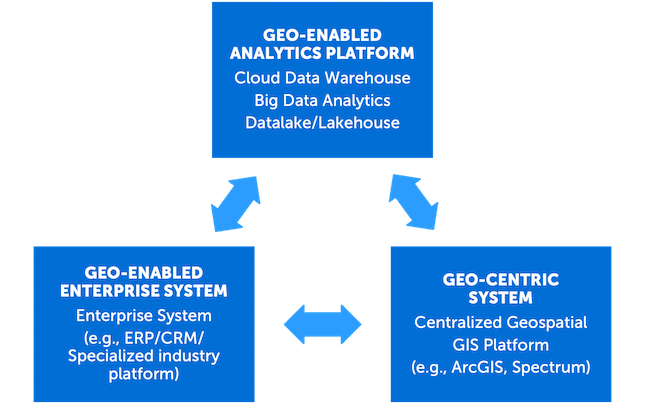
Business Agility
As a company progresses in their digital modernization journey, many are migrating their data warehouses to cloud-native and big data analytics platforms such as Databricks, Snowflake, Google Big Query, and others, with the goal of achieving more business agility. IT departments need to demonstrate time-to-value, process elasticity for infrastructure cost savings, and access to advanced platforms and tools that can manage the deluge of data. This rationale is based on their ability to adopt advanced analytics and machine learning for a variety of use cases, whether for large batch processing or real-time operational model integration.
These next generation, geo-enabled platforms, like Databricks, that adopt the lakehouse paradigm constitute a very powerful tool not only in the arsenal of the data scientist, but also for the CIO that wants to achieve faster results for advanced use cases. A lakehouse offers the advantages of both a data warehouse and data lake by providing the ability to run analytical queries against all data types (e.g., structured and semi-structured) without the need for a traditional database schema. According to Databricks, storage is decoupled from the computer environment which utilizes separate clusters. Being able to spawn a Databricks cluster in minutes and execute a model at scale, without having to maintain the underlying hardware and software architecture, brings both autonomy and agility to business processes.
Exploiting the Inherent Advantages of Geospatial Data and Technology
These environments also need to be able to exploit the geospatial attributes within a lakehouse architecture and leverage their distributed processing and scalability. Whether it is customer records, company assets, tracking information such as GPS or IoT traces, companies will accumulate terabytes of data, almost all tagged with a location coordinate, such as a latitude and longitude.
While some vendors that adhere to a lakehouse architecture support some geospatial querying functionality, they are not on par with the breadth of capabilities offered by a traditional geocentric or GIS platform. While moving data back and forth using GeoETL between the lakehouse and the GIS system may work for smaller data volumes, it does not for higher data velocity and volume. As data volumes increase, moving data between computing domains rapidly becomes an unsustainable option, from both a data science and IT perspective.
Fortunately, some of these platforms do have a level of built-in geospatial capabilities. Geospatial vendors are starting to offer extensions, for example CARTO’s Big Query UDF extensions, or Precisely’s suite of cloud native SDKs that support multiple technologies such as Databricks, Snowflakes and Kubernetes. These vendors usually provide vector and sometimes raster geospatial capabilities, but more geospatial functions like geocoding, address validation, and routing, are being integrated into these types of environments. Such integration allows both performance and flexibility thereby avoiding data transfer and, at the same time, leveraging the power of the cloud’s underlying distributed processing.
Reality Check
Today, many BI solutions (e.g., Tableau, Cognos) include map visualization and at least rudimentary spatial analysis as standard functionalities. Popular databases (e.g., Oracle, Microsoft Azure, IBM Db2) have extensive support for geospatial primitives and coordinate systems, as well as spatial analysis. With 85% of enterprise data having a geospatial component, there is an undeniable need for both, geocentric platforms, like GIS, geo-enabled BI solutions that support end users, and elastic data lakehouses needed by corporate IT environments.
Geospatial technology and data, therefore, has become an essential tool in the business user’s toolbox. Geospatial may require an engagement with a company with the knowledge and experience to tackle both data use cases and the solution architecture, that sit at the crossroads of advanced geospatial analytics and modern cloud architecture. Korem is your cloud-fluent, geospatial technology integrator.