Four Instances Where Mobility Data Offers an Edge in Retail
April 25, 2024
Retailers, fuel retailers, and commercial real estate companies leveraging mobility data, especially vehicular traffic data, have a distinct advantage over competitors in making better business decisions. This type of data provides hyperlocal insights for site selection, including white space analysis and cannibalization analysis.
However, creating maximum value from detailed mobility data requires geospatial expertise. An approach tailored to your industry, company size, unique challenges, and business goals will always be more powerful than a one-size-fits-all approach. These factors all determine which data you should use and how to implement it in your organization.
In light of our experience, we’ve identified four instances where a generic approach failed to deliver anticipated results.
- Defining trade areas
- Road segment selection strategy
- Intersection screening for site selection
- Understanding consumer behavior beyond traffic
Korem’s expertise then ensured organizations unlocked the complete potential of their data investments, according to their unique requirements and objectives.
1. Defining Trade Areas
Defining the trade areas of existing or potential retail sites is not as simple as it sounds, but it’s crucial for site analysis. It can be used to predict sales revenue, optimize store planning and networks, replicate store success, and even enhance marketing efforts. Different approaches exist depending on the chosen sets of parameters, like time, distance, and boundaries, and each one generates different sizes and shapes of trade areas.
On one hand, defining trade areas based on a chosen distance, e.g., a one-mile radius geographic area, generates homogeneous trade areas that facilitate comparison amongst sites. However, it can introduce noise in the data since it could include boundaries that are not relevant or accessible for the analysis.
On the other hand, defining trade areas based on driving time (isochrones), e.g., the time it takes potential customers to get to the retail store from home or work, generates a more coherent trade area. However, its irregular size and shape might be a bit more difficult for comparison between multiple retail sites.
A further approach involves taking into consideration existing boundaries, which can include geographic barriers such as rivers and highways, ZIP code boundaries, designated market areas (DMA), and political and administrative boundaries like counties, to generate custom and more precise trade areas.
But what is the best solution between these approaches? Again, it all depends on each case. At Korem, we specialize in analyzing consumer data in relation to the traffic in trade areas of different sizes and types. This enables us to have a complete portrait of customers’ traveling and shopping habits based on a wide range of characteristics.
In this one real example, Korem’s methodology achieved an increase of up to 50% in the correlation between captured traffic and our customer’s metrics.
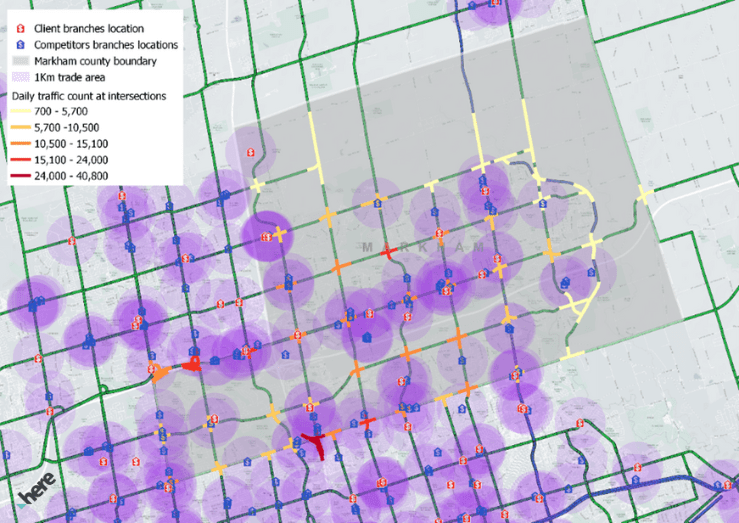
Example of trade areas defined by distance
2. Road Segment Selection Strategy
Korem exploits multiple road attributes to have a more accurate understanding of traffic volumes based on the time of day and the geographic realities of the territory.
Firstly, we take into account the different types of vehicles in traffic and how it can influence results. For example, trucks have limitations for transit in suburban areas.
Secondly, we clearly define which road segments will be included or excluded from a trade area. Using road function classifications, as well as granular traffic data, helps fine-tune the selection by visually identifying the road segments that generate noise and dilute the quality of the aggregated data during the analysis. For example, in a data delivery use case, our team realized that parking lots within our client’s trade area generated a lot of traffic counts without being relevant to the analysis.
Thirdly, using street attributes, we select the common characteristics of road segments to be excluded in order to develop a new data processing rule.
Here’s an example of a map with different trade areas, for which traffic data has been aggregated by road classification segments and a one-kilometer radius.
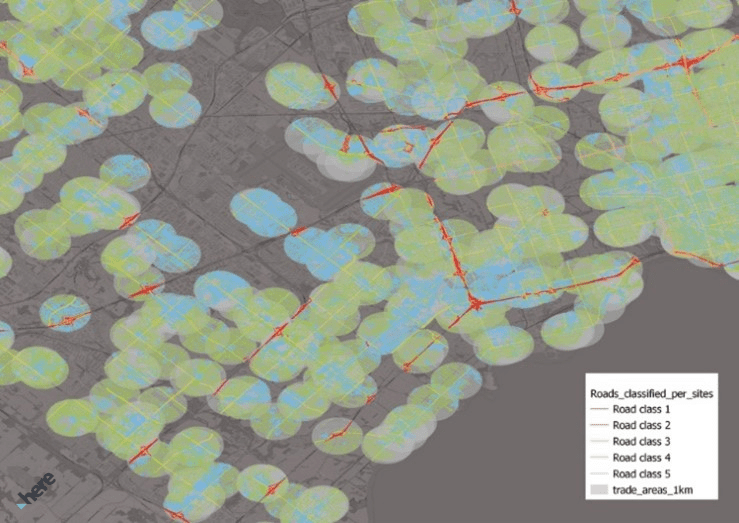
And here’s what traffic data with daily aggregation sites looks like:
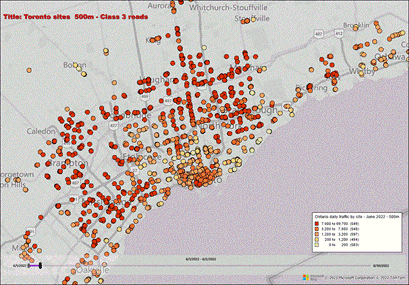
3. Intersection Screening for Site Selection
Analyzing traffic at different road segments with historical traffic data (ideally over 2 years to account for seasonality) will identify the busiest segments with potential for new retail stores and commercial real estate developments. To avoid cannibalization, we can analyze this data with your existing store locations and your competitors’ locations.
One of Korem’s clients asked us to produce a method to extract traffic data around street intersections to identify high-traffic areas and determine the best potential locations for future branch openings. In the process, we identified a problem that could have made the analysis inaccurate. Some points were identified as intersections, when in fact it was not intersections of two or more roads in a perpendicular direction, but rather bifurcations or junctions of roads such as these:
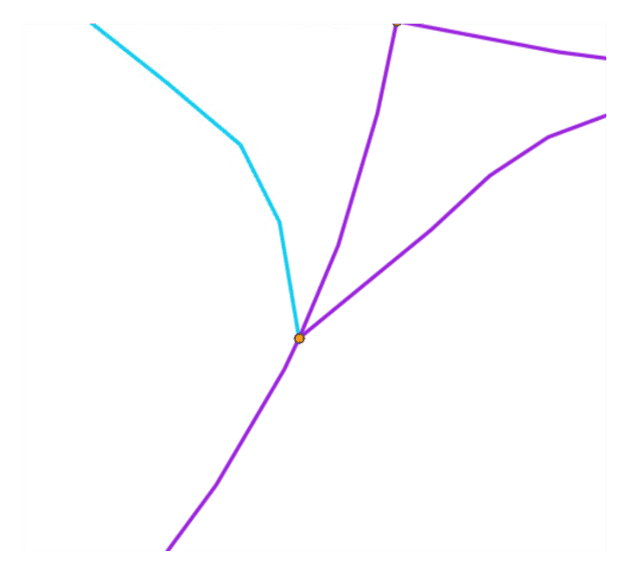
Korem then developed a model using road network attributes and GIS geometric operations that would filter out these kinds of bifurcations and not count them as intersections.
4. Understanding Consumer Behavior Beyond Traffic
Using complementary data sources like demographic data, foot traffic data, and cross-shopping information contributes to enriching the decision-making process by considering other variables. Korem’s facilitates this process by creating customized dashboards including all relevant data, improving the visual representation of the trade area analysis.
For example, footfall traffic provides a better understanding of consumer behavior and delivers a hyperlocal view of the propensity and frequency of visits to local stores. However, foot traffic data, which is derived from phone data, is a bit more challenging from a legal and technical point of view. It’s therefore important to deal with a team of experts that has in-depth knowledge of the terms and conditions of data contracts and licensing agreements.
When a One-Size-Fits-All is Not Always the Answer
Using a one-size-fits-all approach to mobility data is most often just the beginning of a data-driven journey. Without a sophisticated strategy to truly capture the relevant traffic segments around your sites, the value of traffic data is not optimally exploited.
There is therefore real value in making an exhaustive analysis of the correlations between different data and key business indicators. Investing efforts in understanding traffic patterns in trade areas can truly improve the value of traffic data and ultimately help organizations make better business decisions regarding their site analytics strategies.
Korem has demonstrated time and again the potential of combining detailed traffic data with our unique geospatial expertise. If you want to explore solutions tailored to your unique needs or even access a live demo, book a call with our experts’ team!