The Importance of Data Integrity Before Launching an Artificial Intelligence (AI) Project
Recent years have seen an increase in the popularity of artificial intelligence (AI) and, by extension, machine learning (ML). Together, AI and ML make sense of the increasingly large quantities of data available to companies, helping them to model, predict, and then prescribe a suitable approach to solving problems of various kinds. For instance, they can be used to improve route planning and delay forecasting for time-sensitive logistics processes, such as fleet management, as well as facilitate site selection to maximize sales according to local demand and competition.
According to Gartner, over the past few years, the number of companies adopting artificial intelligence leaped by 270%, and to date, over 37% of them have implemented the technology in one form or another. By 2028, the AI market is expected to have reached USD 641.3 billion, an absolutely spectacular increase from 2020, when the market was valued at USD 51 billion.
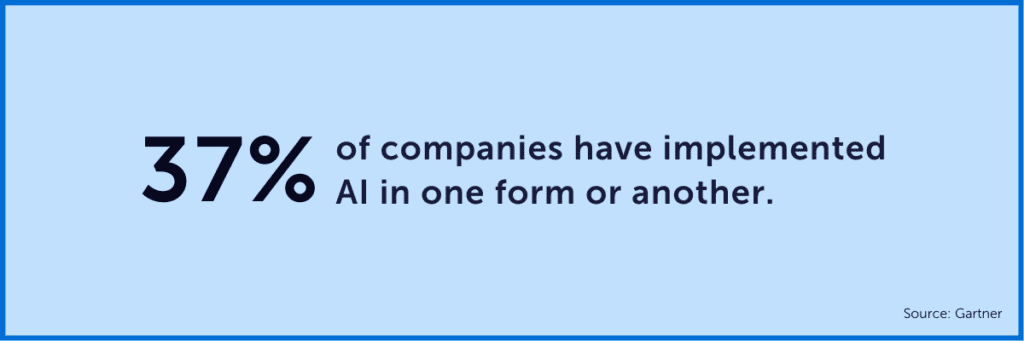
However, AI and ML can easily end up in the “shiny objects” category—something that appears promising and exciting at first sight but turns out to bring no added value whatsoever. There’s no doubt that when used properly, these technologies can offer a significant advantage, unlocking new opportunities, generating major operational efficiency gains, increasing the speed and effectiveness of the decision-making process, and improving the user experience in terms of products and services.
But unfortunately, a number of companies have succumbed to the temptation of investing in these sophisticated new technologies when they—or rather their data—weren’t necessarily ready to make such a big jump. Consequently, before they can be in a position to really benefit from AI and ML, businesses must first verify the integrity of their data.
Issues Organizations Have With Data
Some companies embark on artificial intelligence projects on a whim, thinking—wrongly—that success depends solely on the technology itself or the supplier with whom they’re doing business. In a hurry to integrate the new technology into their processes, they tend to get ahead of themselves, not taking into account the state of their data environment.
Organizations often fail to realize that data warehouses are the beginning and end of a process, and that they don’t have what should be in the middle, transforming raw, unlabeled data into usable information. This middle layer, dedicated to the normalization of said data and considerably less popular than AI or ML, is often unknown territory to the decision-makers in charge of IT strategy. So, the organizations in question have to make do with unnormalized data stored in an unsuitable format, making accessing and using the data a far more complex task.
Of course, the difficulties generated by unnormalized data only get worse when new sources of external data are added, as multiple data mean multiple problems. The companies involved also run the risk that the freshly acquired data may be of poor quality. As they don’t have an effective data processing system in place, data scientists have to spend, on average, 80% of their time collecting, cleaning, normalizing, and organizing data rather than contextualizing and enriching it. What’s more, 76% of these specialists see preparing and managing data as the least enjoyable part of their work.
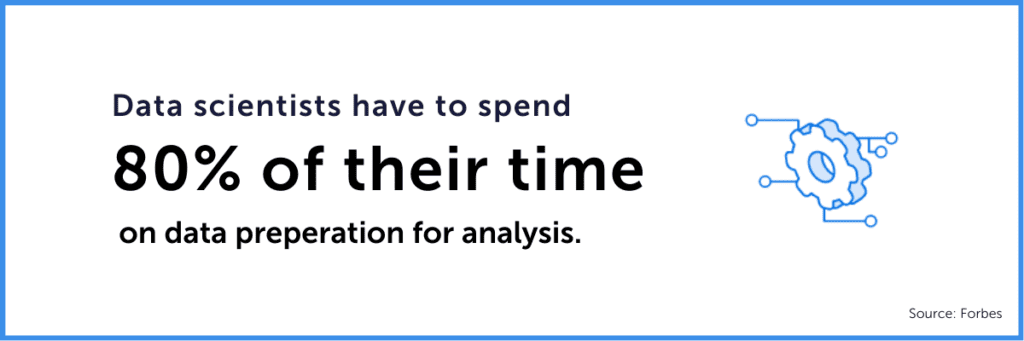
And that does not take into account that some companies are facing a glaring lack of resources and expertise in their teams due to the worker shortage and the costs associated with upskilling. This situation prevents them from fully exploiting emerging technologies.
Sooner or later, AI or ML initiatives end in failure or don’t achieve their full potential owing to a lack of operational preparation and gaps in the raw material, i.e., the data itself.
Preparing the Data Environment Properly
Before succumbing to the temptations of sophisticated technological solutions, companies would be far better off solving the problems arising from the varied nature of their data by investing in its integrity, i.e., in its accuracy and validity throughout its life cycle.
“Data quality and integrity are essential for ensuring the overall consistency of the data within the lakehouse for accurate and useful BI, data science and machine learning,” explains Databricks.
Fortunately, there are several ways of getting around the problem.
1. Company-Wide Data Integration
First, suitable warehousing of a variety of data is key to solving speed and volume issues, which have a far-reaching impact on effective data integration within a company. The aim here is to transform similar data arriving from several different places into a common, standardized form and then assemble it all in a single data lake.
Then companies would be able to create their own, standardized databases on a single company cloud data platform, such as Databricks, Snowflake, Google BigQuery, AWS Redshift, or Azure Synapse, to name but a few. While the availability of cloud data warehouses, big data analytics, and artificial intelligence and machine learning platforms undoubtedly make the “technical bit” a lot easier, everything still depends on data quality.
With this type of platform, companies know how and where their data is stored, in what format, and in which volumes. Even better, as all the data is assembled in one place in an entirely automated environment, it is easily accessible, significantly optimizing its use.
2. Data Accuracy and Quality
Next, it’s crucial to integrate data quality alerts and regular accuracy checks, in order to guarantee the viability and stability of the artificial intelligence algorithms. Having access to reliable data is required for reliable, strong AI. If AI is unreliable, companies cannot possibly benefit from its promised value, in the form of automated, tried, and tested commercial decisions. Currently, 84% of CEOs are worried about the integrity of their data. This figure is particularly alarming when you consider that most of their decision-making procedures are based on that very data. One Gartner survey has even found that organizations lose USD 15 million per year owing to poor quality data.
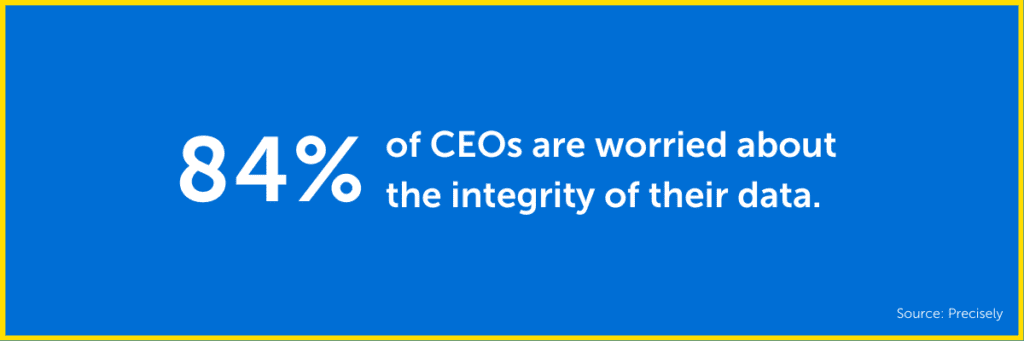
What makes data accuracy even more important is that machine learning algorithms are particularly vulnerable to unreliable data. This is because, since these algorithms use large quantities of data to adjust their internal parameters and distinguish between similar models, even small errors can lead to large-scale mistakes in the system’s results.
The quality of a company’s data is therefore directly correlated to its results and credibility. What’s more, when less time is wasted sorting third-party data, data scientists can use their time more profitably by concentrating on value-added tasks.
3. Data Contextualization and Enrichment
Finally, geospatial technology is obviously a major tool in any data scientist’s arsenal. Geocoding, geospatial players, and enrichment data all make it possible not just to establish links and relationships between various components, but also to validate data and put it into context.
More often than not, the addition of further attributes by means of geo-enrichment is vital in terms of feeding artificial intelligence and machine learning models. By enriching data with extra information, such as geographical, mobility, or demographic data, it’s easier for companies to contextualize the environment, which in turn means they can validate and correct their data and ultimately increase the effectiveness of their predictive analyses.
Advantages of a Geospatial Company Like Korem
For organizations, acquiring the necessary in-house professional expertise to quickly operationalize data and then successfully integrate artificial intelligence, can turn out to be an expensive, complex, and energy-intensive operation. As a rule, artificial intelligence is not an end in itself but rather a way of achieving a business objective. One can think, for example, of modeling for decision-making or prescribing and automated decision-making to increase organizational efficiency and performance.
Before embarking on a costly AI or ML initiative, not only should companies make sure their data is ready to be used, but they should also explore how contextualizing and enriching this data with external quality data might simultaneously improve its usability and geospatial capabilities. Then companies would be able to complement and improve their modeling and automated decision-making algorithms.
If you put your trust in Korem’s expertise in data integration, geocoding, external data enrichment, geospatial analytics, Data as a Service (DaaS), and spatial data science, you will quickly make sure that you are not under-using the options that ensure maintaining data integrity—which is a deciding factor when it comes to the success, return on investment (ROI), and risk reduction associated with your artificial intelligence initiative.